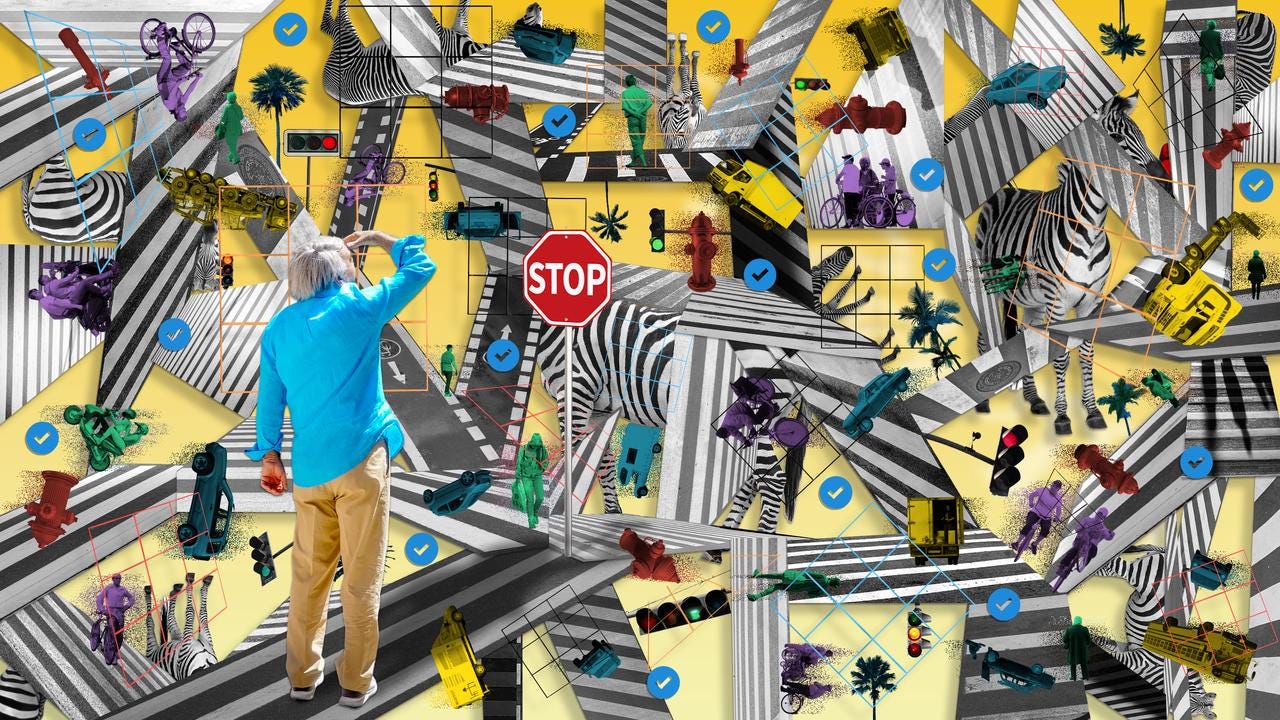
This year, almost half the world’s population will have the chance to vote. Over 50 countries, including India, the United States, Indonesia, Russia and Mexico, will go to the polls, deciding the future of more than 4.5 billion citizens.
As has been said so many times, one of the biggest contemporary dangers for democracy is misinformation and lack of media literacy.¹ Today’s press is acting in an age of dubious credibility. Misinformation has spread beyond shady conspiracy blogs, and into several mainstream media channels that historically have a high level of credibility and journalistic integrity such as The Washington Post,² The New York Times³ and The Guardian.⁴ Not only that, the emergence of social media as a primary news source has opened the floodgates for misinformation.⁵
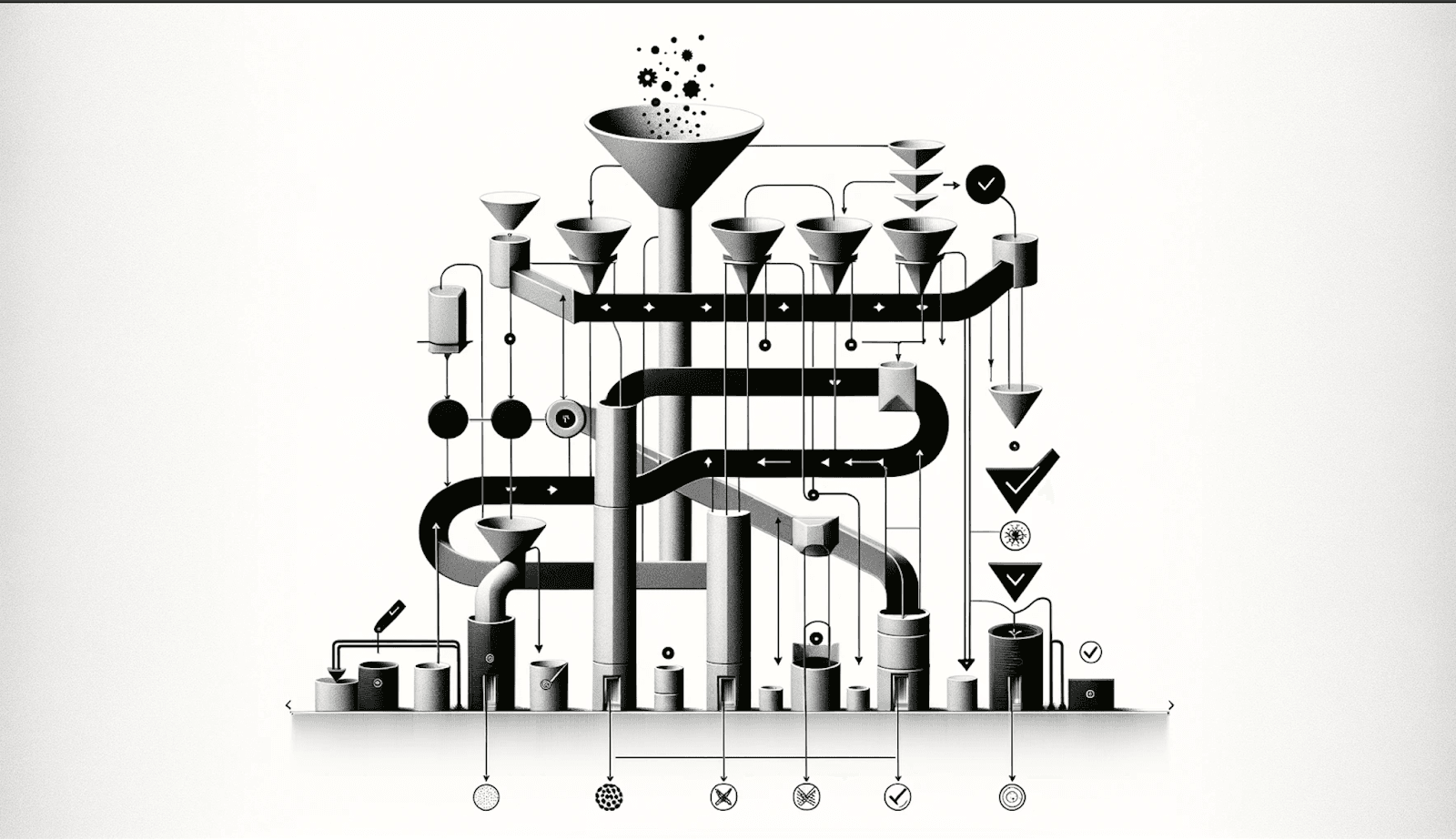
The rise of fact-checking industry & algorithmic faith
This is, of course, a huge problem not only for democracy but for our general understanding of reality. How can we make our own decisions in a rapidly changing world that is only seen through the lens of biased media? The answer has been a global increase in fact-checking organizations and companies that help newsrooms, policymakers, and the general public understand and make distinctions between fact and fiction.
Within this new industry, a lot of technology is already emerging. Startups and organizations like Claim Buster, Africa Check, Chequeado, Newtral or Verificat are already working on human-supervised fact-checking platforms, which have become essential tools for maintaining credibility in politics and journalism. There are interesting companies like Full Fact, playing between automating statement monitoring in politics, but what I’m personally afraid of are the growing calls for automatic, algorithmic fact-checking. You can see organizations such as Fact Instect going in that direction. This is a solution to a problem that creates a new one; it means we are delegating the definition of “truth” to an algorithm, which is dangerous for several reasons. Let’s take a look at three of them in more detail.
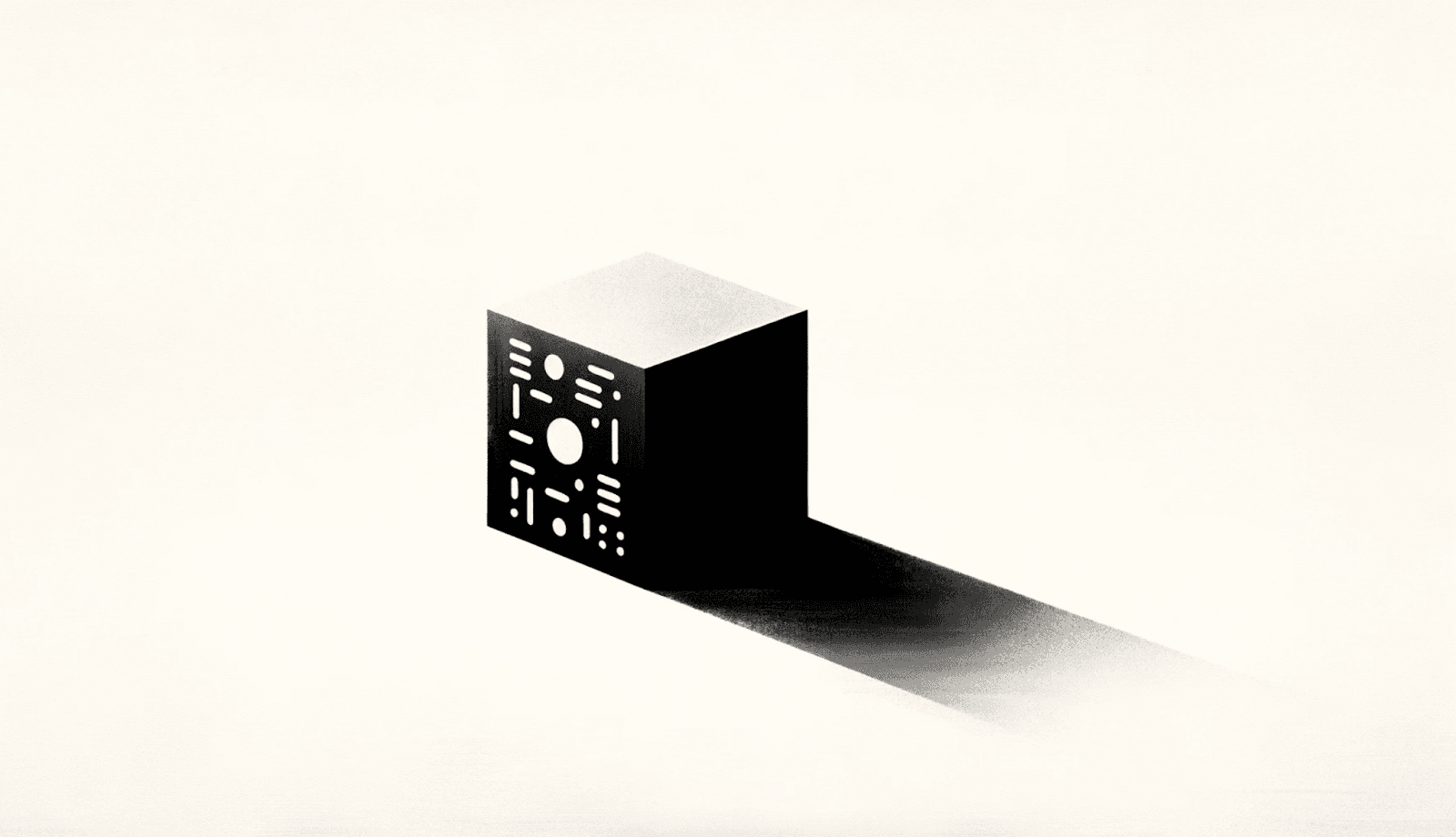
1. Truth goes hand in hand with transparency
Transparency is key in media newsrooms; a recent study on ResearchGate⁶ demonstrated that news organisations critically enhanced their credibility by being verbal about the journalistic process they were following. On the other hand, Large Language Models (LLM) are often referred to as black boxes because this technology is essentially programming itself, creating a distinct lack of transparency. Through this process, it often learns enigmatic rules that no human can fully understand. This was demonstrated to the world when Google’s AlphaGo, a type of LLM, made a winning move against go champion Lee Sedol that left observers, including the team that created the model, puzzled. Despite the potential to examine AlphaGo’s parameters, the vast and intricate network of connections within makes it almost impossible to understand the rationale behind its decisions. This technology is, therefore, opposed to transparency by nature. If you don’t understand the process by which a piece of tech gives you a result, you effectively place blind faith in it.
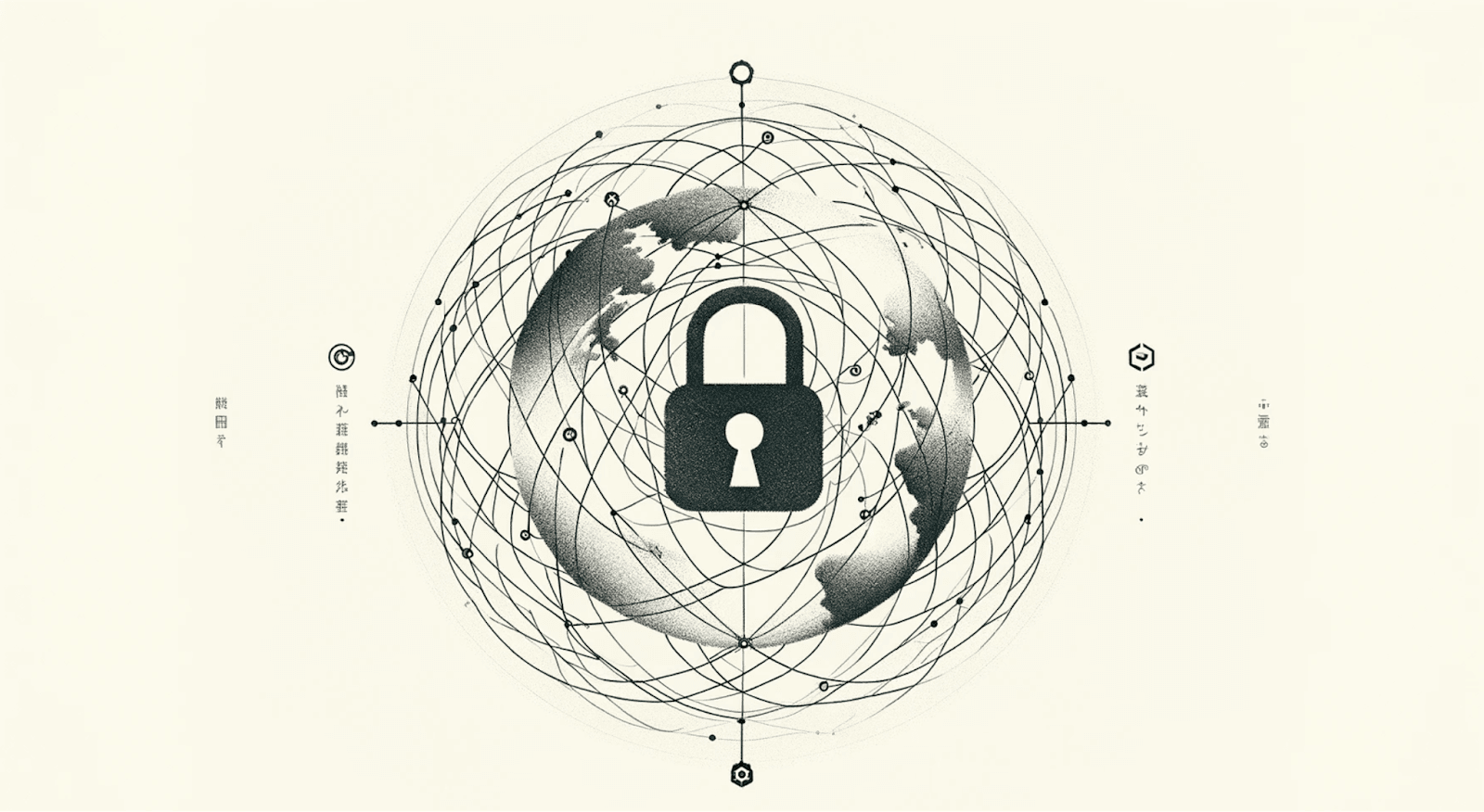
2. Truth can not be owned
Most of the AI models we use today are owned by private companies, and as we have seen in the past, there will eventually come a time when these companies’ interests do not align with those of their users. There are few things that can damage the credibility of a news outlet more than public and private conflicts of interest. The idea that the “press is controlled by the state” or “press has been bought by big corporations” is not new, but now imagine that these powers have not only bought the hand that writes the news but also the software that filters it.
The 2016 Brexit vote in the UK and the chaotic U.S. presidential election showed us that algorithms and social media companies can have a huge influence on the way we decide to vote. A study in 2016,⁷ which looked at 376 million Facebook users and their activity with more than 900 news sources, revealed that people usually look for news that confirms their own opinions. Social media platforms cater to this confirmation bias to encourage greater user engagement at the expense of accuracy and actual facts. Today, we are in front of an even bigger problem; what is at stake now is not visibility, but truth.
3. Unhealthy dependencies
The third reason for concern is that an over-reliance on AI fact-checking could diminish our capacity for critical thinking, making society more susceptible to misinformation when AI guidance is unavailable. Dependence on AI for fact-checking, at its core, is an issue of cognitive outsourcing. Just as we rely on calculators for arithmetic⁸ ⁹ or Google Photos to remember what we did last weekend¹º ¹¹, turning to AI for fact verification could lead to a ‘use-it-or-lose-it’ scenario for our cognitive capacity for critical thinking and discernment.¹² Like a muscle that atrophies from disuse, our collective ability to critically evaluate information, to read between the lines, and to understand the subtleties of argumentation and evidence could diminish. This is not just a loss of individual capabilities but a societal shift towards increased vulnerability to misinformation, propaganda, and demagoguery. Like drivers who follow GPS directions with blind faith, never learning the route, society could become lost in misinformation without reliable software, unable to navigate the landscape of truth unassisted.
Beyond these three reasons, we find that fact-checking with contemporary AI models is a bad idea for numerous other reasons. This is due to intrinsic biases, the potential for misinterpretation or the legal and accountability issues embedded in these models. There’s already a paper that documents how fact-checking with AI could actually undermine human discernment of truth.¹³
Don’t lose hope just yet…
Natural language models can change many things, and through a more interdisciplinary approach, we can discover many interesting ways to use them. Today, they’re mainly used to substitute, automate, or optimise, but there are many more than these three approaches to explore. We need to reframe these tools in the space of media literacy and critical thinking; forget about summarising; let’s focus on how these tools can help us be more critical of the way we experience information.¹⁴ Let’s not delegate our beliefs to an algorithm. Let’s make these tools work for us so we can be more adept at identifying potential misleads, biases or manipulations. We need to create tools that can help us be more independent, critical thinkers by ourselves. Technology should enhance our critical thinking, not replace it.
The challenge, then, becomes one of balance. How do we apply the undeniable benefits of AI to manage a deluge of information while preserving and enhancing our innate human capabilities? This dilemma touches on themes explored by thinkers like Shane Parrish of Farnam Street, who advocates for mental models that help us understand the world. He suggests a need for an educational paradigm that values not just the acquisition of knowledge but the cultivation of wisdom and discernment.
In addressing this challenge, we must also confront the philosophical question of what it means to know something in the age of AI. Is knowledge merely having access to verified facts, or does it encompass the ability to engage with, question, and integrate those facts into a coherent understanding of the world? The answer to this question will shape not only our educational priorities but also the future of our engagement with technology and information.
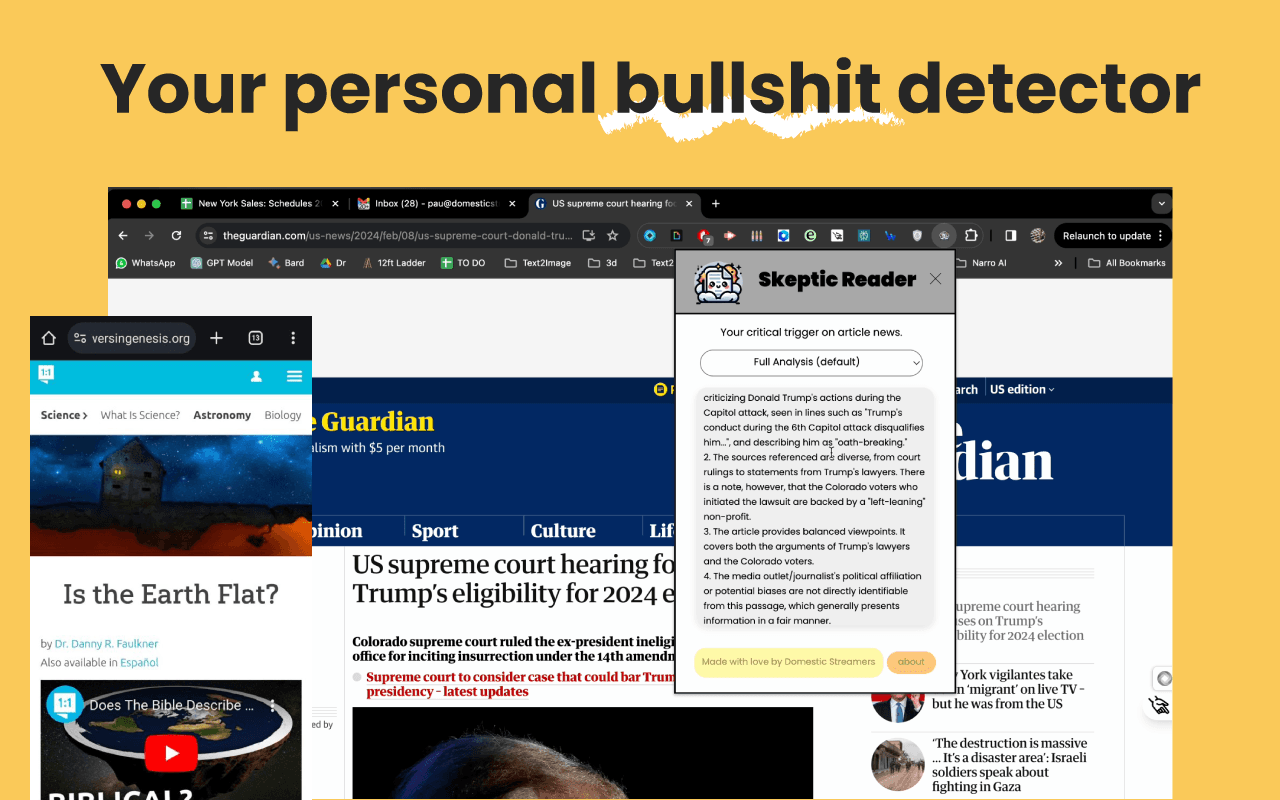
At Domestic Data Streamers, we wanted to explore these ideas further, so we created an experimental piece of software. SkepticReader is a free plugin for Google Chrome browsers that reads the news and generates a critical review of it, identifying logical fallacies and observable bias and giving counterarguments to each piece of the news.
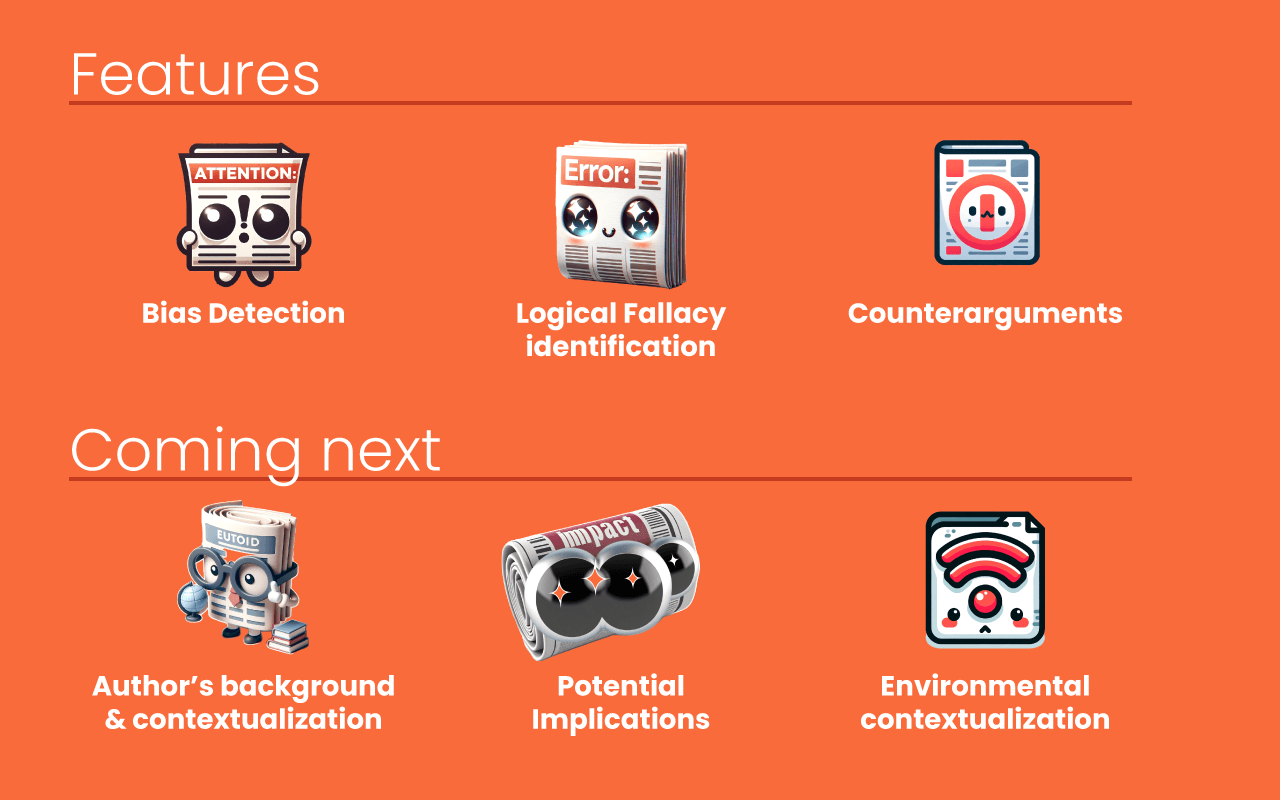
The goal is to cultivate a critical gaze as we read and process information without actually creating a dependence on technology to determine if something is true or not.
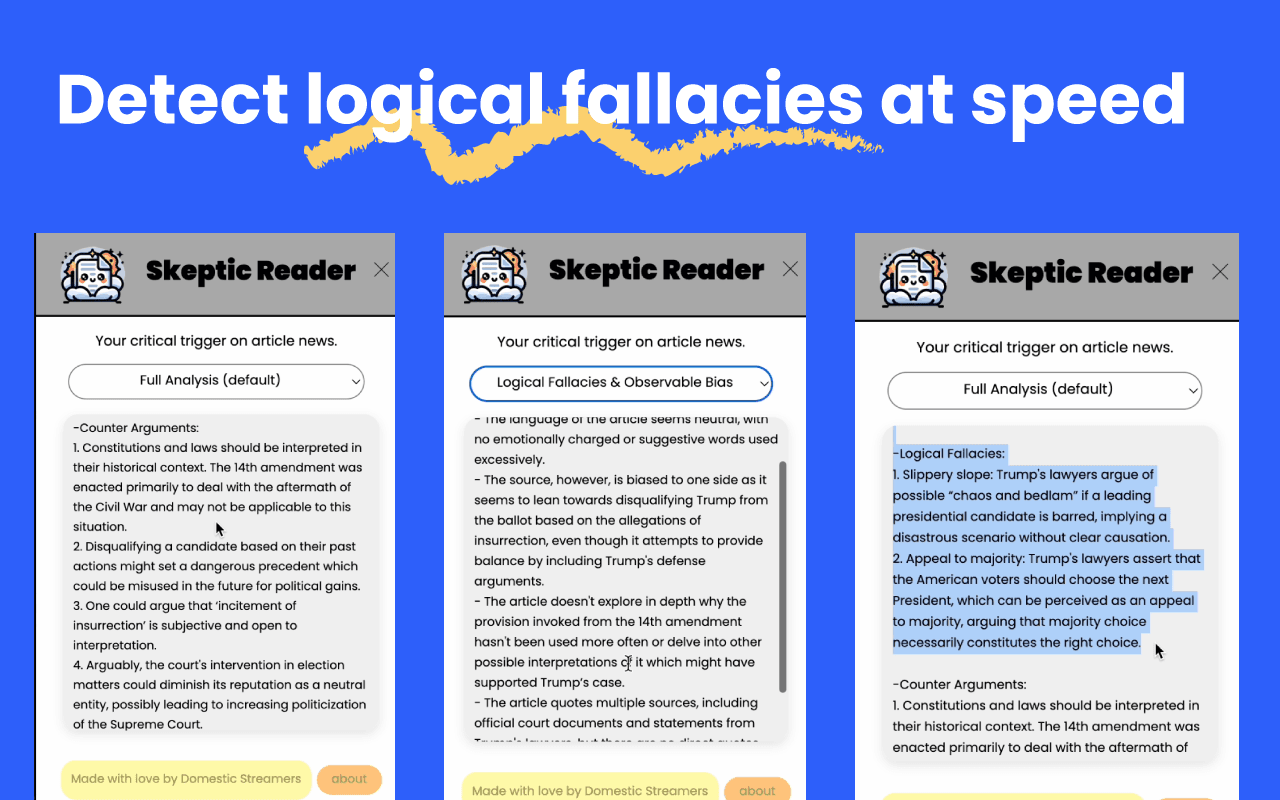
For this experiment, we have decided to use the most mainstream AI models and browsers for greater user accessibility. The underlying models we are using are OpenAI GPT3.5 and GPT4, which, over our last year of research, have proved to be really bad at fact-checking but very good at detecting bad reasoning skills, finding patterns in biased language and detecting logical fallacies.
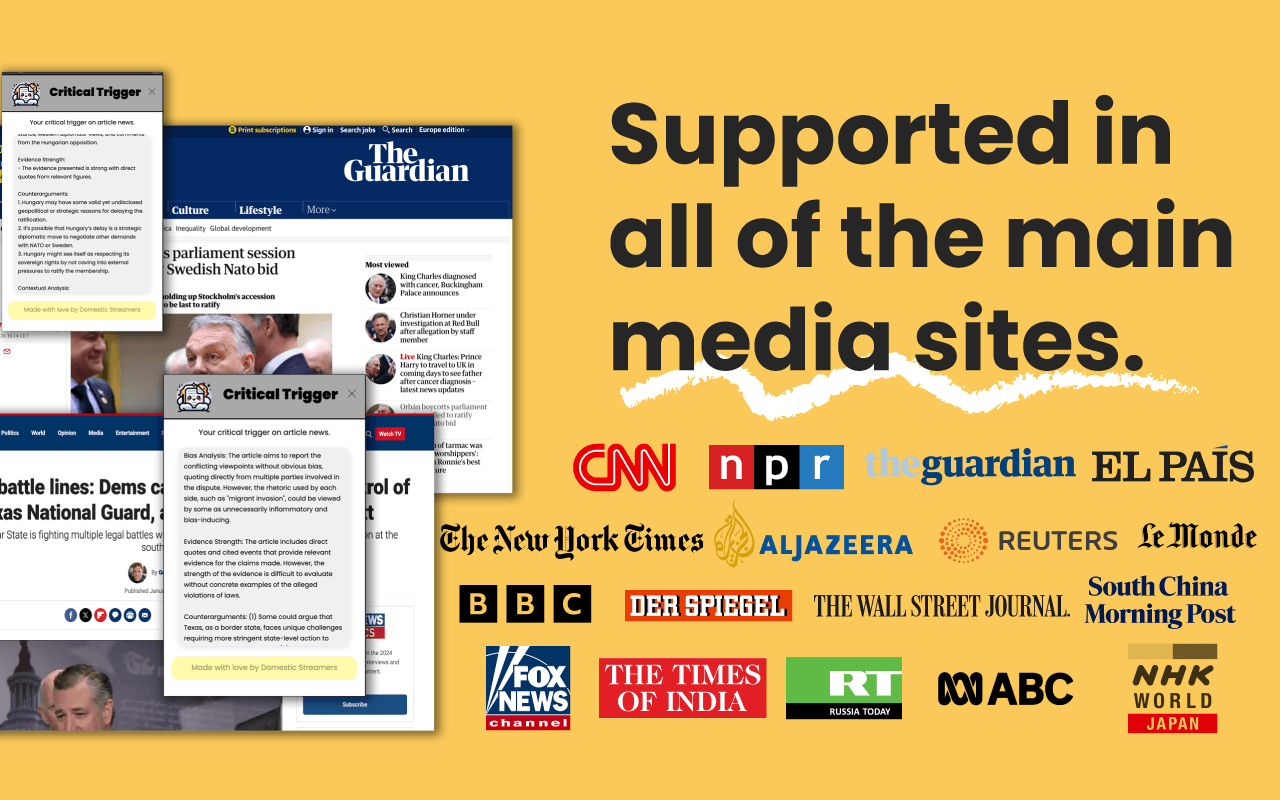
For the browser, we decided to create it for Google Chrome as it is the most popular browser, with 63.6% of the market share as of 2023 and 3.46 billion users worldwide. The second most used was safari, but with only 19% of the market share, it would have made little sense.
The evolution of AI in media opens a broader debate about the nature of truth and knowledge in the digital age. It challenges us to reconsider what it means to be informed and how to best equip ourselves to discern truth in an increasingly complex information ecosystem. In this context, the role of GEN-AI needs to be a facilitator rather than a gatekeeper, prompting a shift towards a more proactive, engaged form of media consumption. As we look to the future, the task is clear: work towards a GEN-AI that empowers individuals, strengthens democratic processes, and fosters a culture of critical engagement with the information that shapes our world instead of substituting our basic discerning capabilities.
You can start using this software here: https://www.skepticreader.domesticstreamers.com/
Further research
With the aim to keep going with some of the main questions deployed through this article, we will keep on our work following some of these research questions:
- How does the plugin impact users’ ability to identify logical fallacies and biases in news articles? This question aims to measure the effectiveness of the Skeptic Reader in enhancing critical media literacy among its users.
- What are the differences in perception of media credibility before and after the use of the plugin among various demographics? This question seeks to understand if Skeptic Reader influences users’ trust in the media and whether this effect varies across different age groups, educational backgrounds, or political affiliations.
- What is the accuracy of the plugin in detecting logical fallacies and biases compared to expert human analysis? This question aims to benchmark the plugin’s performance against that of human fact-checkers or critical thinkers.
- How does reliance on the plugin for critical analysis of news affect users’ own critical thinking skills over time? This explores whether the plugin serves as a cognitive crutch that diminishes users’ abilities or as a tool that fosters and enhances critical thinking.
- In what ways does the plugin influence the diversity of media consumption among its users? This question investigates whether the plugin encourages users to explore a broader range of news sources or reinforces existing echo chambers.
- What are the ethical considerations of using AI like SkepticReader in media consumption, particularly in terms of data privacy and manipulation? This seeks to explore the balance between technological benefits and the potential for misuse or unintended consequences.
- How does the use of SkepticReader alter the relationship between news consumers and publishers? This question looks at whether the plugin shifts the power dynamics between the media and its audience, possibly leading to changes in how news is produced and consumed.
References
¹ Colomina, C., Sánchez Margalef, H., & Youngs, R. (2021). The impact of disinformation on democratic processes and human rights in the world. Policy Department, Directorate-General for External Policies, European Parliament. ISBN 978–92–846–8014–6 (pdf), ISBN 978–92–846–8015–3 (paper). https://doi.org/10.2861/59161 (pdf), https://doi.org/10.2861/677679 (paper).
² The Washington Post and the Russian Hack of the U.S. Power Grid (2016): The Washington Post initially reported that Russian hackers had penetrated the U.S. power grid, only to clarify later that the hack was not connected to the grid itself but involved a utility company’s laptop that was not connected to the grid: https://www.washingtonpost.com/world/national-security/russian-hackers-penetrated-us-electricity-grid-through-a-utility-in-vermont/2016/12/30/8fc90cc4-ceec-11e6-b8a2-8c2a61b0436f_story.html /// https://www.rappler.com/world/us-canada/157065-russia-hackers-penetrate-us-electricity-grid/
³ The New York Times and the Alleged Approval of Russian Bounties on U.S. Troops (2020): The New York Times reported that Russia offered bounties to Taliban-linked militants to kill U.S. troops in Afghanistan, a story that sparked significant controversy and debate. Subsequent investigations by the Biden administration found low confidence in the bounty allegations, indicating the complexities of intelligence assessments and the challenges of reporting on them. https://en.wikipedia.org/wiki/Russian_bounty_program
⁴ The Guardian and Paul Manafort’s Alleged Visits to Julian Assange (2018): The Guardian reported that Paul Manafort had held secret talks with Julian Assange in the Ecuadorian embassy in London, a story that faced significant scrutiny and for which corroborating evidence has not emerged, leading to questions about the report’s accuracy. https://mondediplo.com/2019/01/10guardian // https://theintercept.com/2018/11/27/it-is-possible-paul-manafort-visited-julian-assange-if-true-there-should-be-ample-video-and-other-evidence-showing-this/ //
⁵ Sadiq Muhammed, Saji K. Mathew (2024, February 18). The disaster of misinformation: a review of research in social media. PubMed Central. https://www.ncbi.nlm.nih.gov/pmc/articles/PMC8853081/
⁶ Masullo, G. M., Curry, A. L., Whipple, K., & Murray, C. (2021). The Story Behind the Story: Examining Transparency About the Journalistic Process and News Outlet Credibility. Journalism Practice, 16(3), 1–19. https://doi.org/10.1080/17512786.2020.1870529
⁷ Schmidt, A. L., Zollo, F., Del Vicario, M., Bessi, A., Scala, A., Caldarelli, G., Stanley, H. E., & Quattrociocchi, W. (2017). Anatomy of news consumption on Facebook. Proceedings of the National Academy of Sciences, 114(12), 3035–3039. https://doi.org/10.1073/pnas.1617052114
⁸ Gratzer, W., & Krishnan, S. (2008). Technology and xx. International Journal of Mathematical Education in Science and Technology, 39, 952–960. https://doi.org/10.1080/00207390802054482.
⁹ Onwumere, O., & Reid, N. (2014). Field Dependency and Performance in Mathematics.. European journal of educational research, 3, 43–57. https://doi.org/10.12973/EU-JER.3.1.43.
¹º Smith, S., & Vela, E. (2001). Environmental context-dependent memory: A review and meta-analysis. Psychonomic Bulletin & Review, 8, 203–220. https://doi.org/10.3758/BF03196157.
¹¹ Ward, A. F. (2013). Supernormal: How the Internet Is Changing Our Memories and Our Minds. Psychological Inquiry, 24(4), 341–348. Taylor & Francis, Ltd. Retrieved from https://www.jstor.org/stable/43865660
¹² Shanmugasundaram, M., & Tamilarasu, A. (2023). The impact of digital technology, social media, and artificial intelligence on cognitive functions: A review. Frontiers in Cognition, 2. https://doi.org/10.3389/fcogn.2023.1203077
¹³ DeVerna, Matthew R., Harry Yaojun Yan, Kai-Cheng Yang, and Filippo Menczer. “Artificial Intelligence Is Ineffective and Potentially Harmful for Fact Checking.” arXiv preprint arXiv:2308.10800 (2023). https://arxiv.org/abs/2308.10800.
¹⁴ Garcia, “Uncertain Machines and the Opportunity for Artificial Doubt.” Medium. (2023) https://domesticdatastreamers.medium.com/uncertain-machines-and-the-opportunity-for-artificial-doubt-5a4e84dd98b6