In recent months, generative Artificial Intelligence models have flooded the mainstream media, with names like Dalle2 and Midjourney becoming familiar references in daily conversation. These models are capable of creating new images, text, or other media that are based on and closely resemble existing data sets.
However, one of the challenges associated with these generative models is their inherent biases, as they are trained on specific datasets that may not accurately represent all populations. In particular, generative models may underrepresent or misrepresent groups from non-Western countries because most of the data that has been fed to them is sourced from western countries. More than half of the data sets come from 12 elite institutions and corporations based in the US territory, Germany and Hong Kong. Very little data comes from Latin America and almost no data from Africa. There needs to be more balance between the source of data and the place it is put to use.
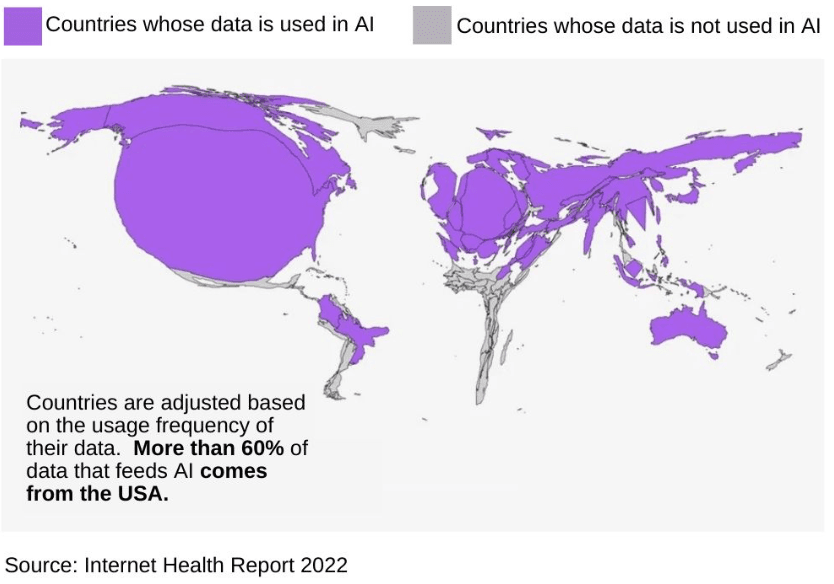
Today, AI tools are in constant use across the globe, but the data they are working from only reflects and represents a handful of countries and institutions.
This quickly becomes evident with a little experimentation, for example, asking DALL E 2 to generate images of: “An old married couple.”
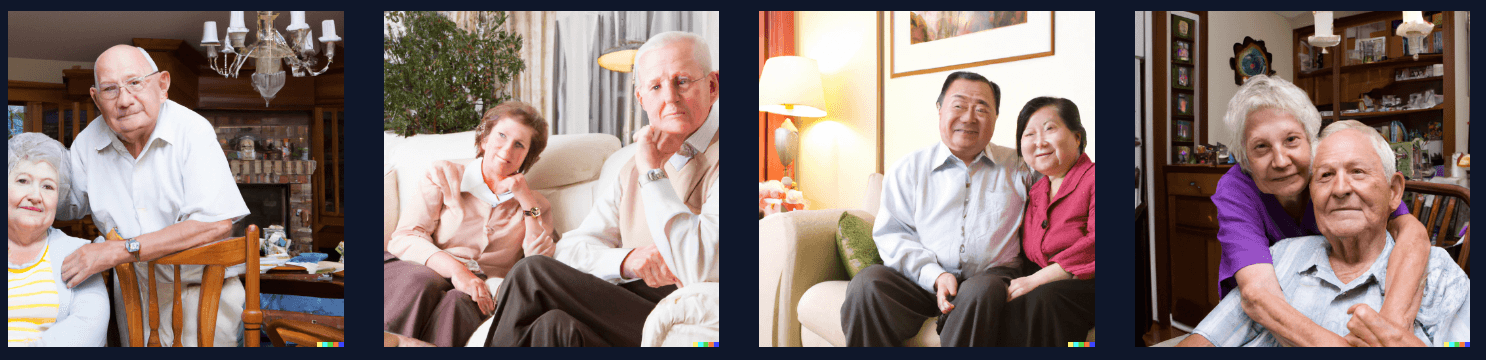
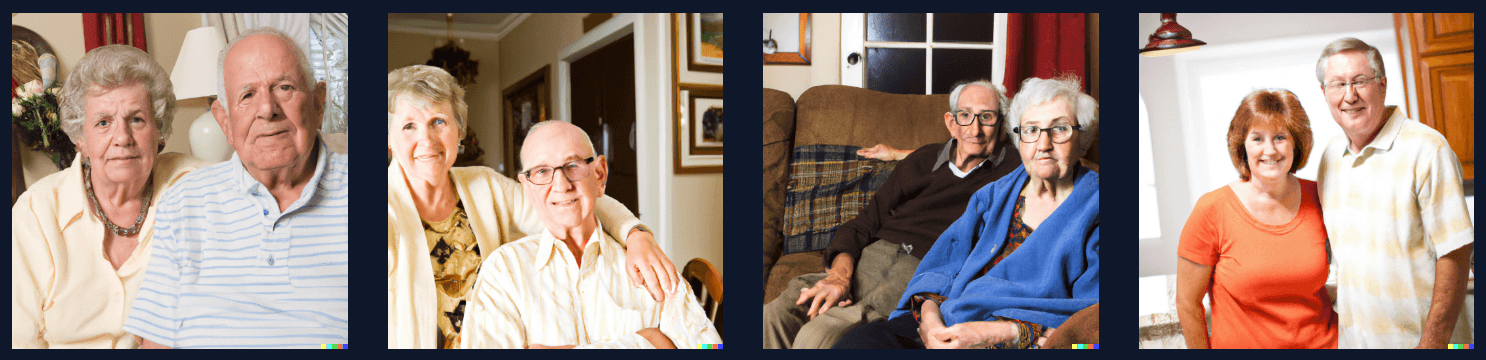
This same request was used in Playground, DreamStudio, Midjourney, NightCafe, Images.AI, Stable Diffusion v1.5, and Krea, and in all the generations only 1 in 8 images was of non-white couples. This is just an example, but many papers have been released on this topic, as well as research and tools like the Average Face for profession.
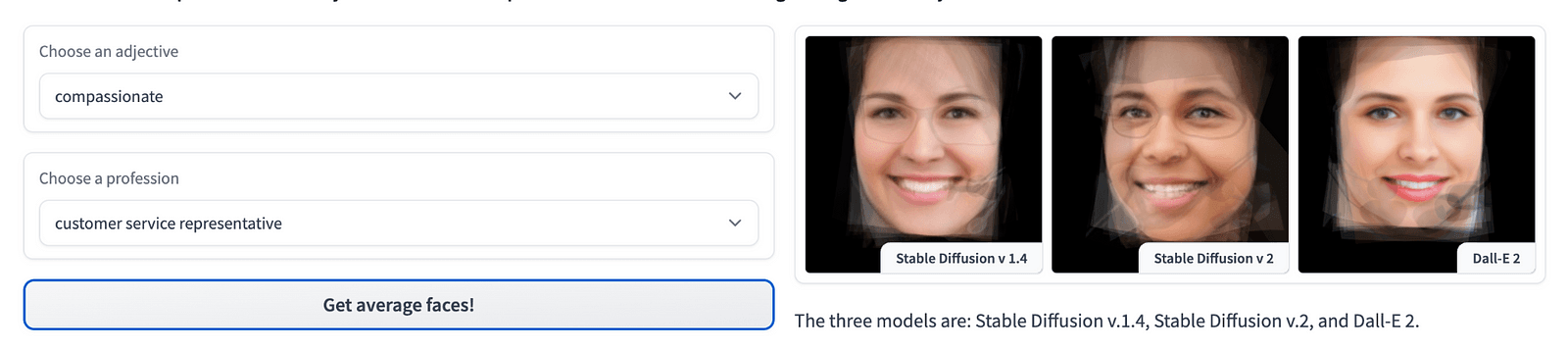
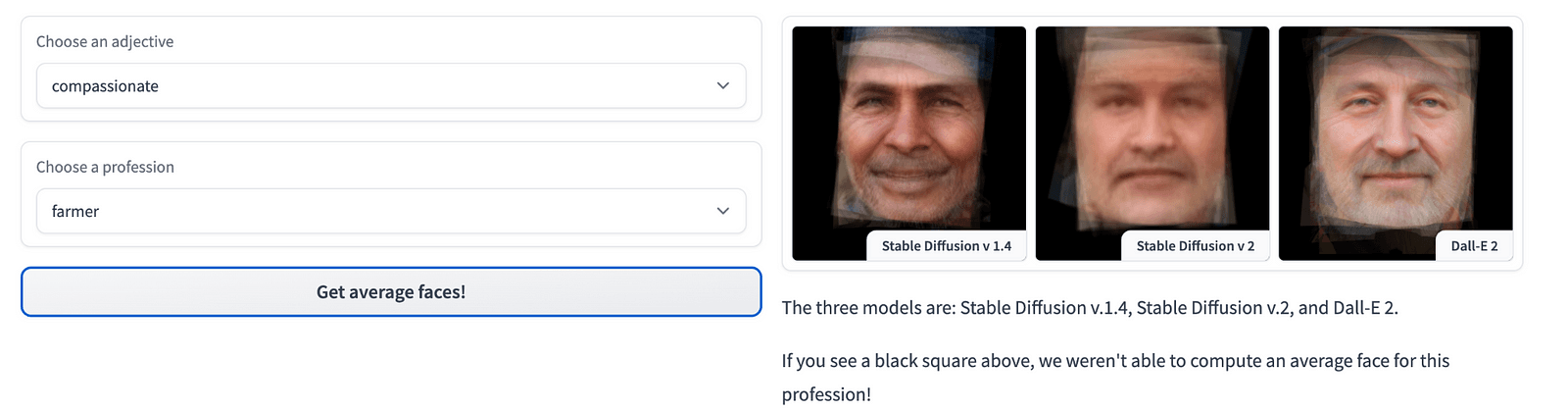
These limitations raise ethical concerns about the use of generative models in various applications. Right now, it’s like letting a kid loose with a paintbrush without giving him the colour red. You will never see red in the final artwork. The same happens with certain ethnicities, fashion styles, architecture, and so on. If it’s not in the database, the algorithm won’t be able to reproduce it, and if these tools are integrated into the mainstream en masse, then these realities could start to vanish from certain collective consciousness and representation.
Artificial Intelligence tools explain themselves as intelligent, and by doing so, they somehow say: “I’m a tool that knows how reality works; let me show it to you. Let me draw a map.” But that’s not true; AI doesn’t know, and neither can it draw truly representative maps of reality.
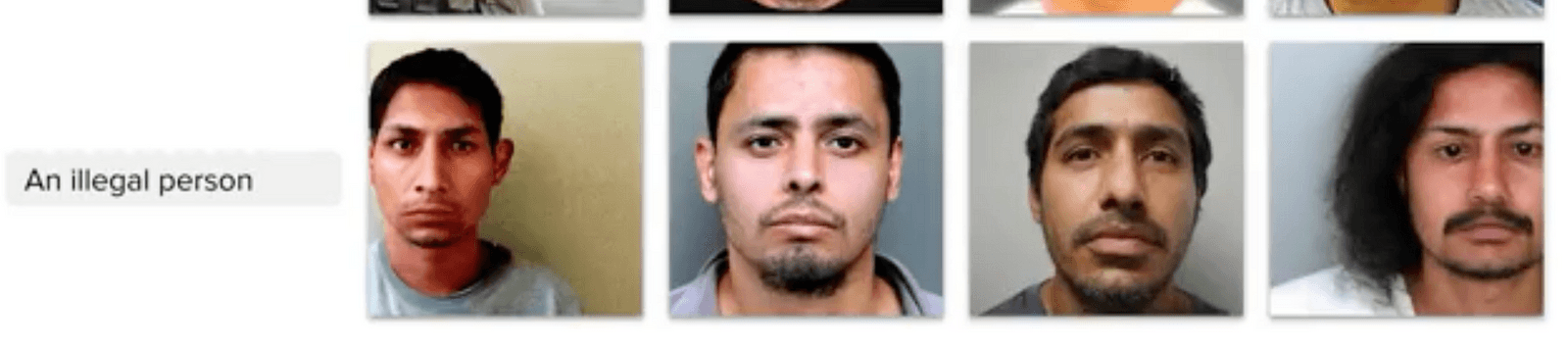
The processes of AI tools are heavily compressed and by doing so, they build up an altered map of reality; like many advanced technologies, they are closed and not open to community negotiation and debate. We want these tools to be open to community access and, more importantly, community consent.
As written by Matteo Pasquinelli at The Nooscope:
Machine learning is a term that, as much as ‘AI’, anthropomorphizes a piece of technology: machine learning learns nothing in the proper sense of the word, as a human does; machine learning simply maps a statistical distribution of numerical values and draws a mathematical function that hopefully approximates human comprehension. That being said, machine learning can, for this reason, cast new light on the ways in which humans comprehend.
These systems have a gigantic inability to recognize the unique, unexpected, and surprising realities that our lives are comprised of, the anomalies that appear for the first time, such as a new joke in a conversation between friends, a metaphor in a new song or an unusual obstacle on the road (a pedestrian with an interesting fashion choice? A plastic bag?). That’s why Tesla and other self-driving companies are having so much trouble with the implementation of these technologies in real-life scenarios because they cannot predict nor IMAGINE things that haven’t happened in the past (in their database). It builds an autocracy of the past. It normalizes whatever structures we have accepted from the database and implements them as all the possibilities you can have in the future.
To address some of these concerns, an experiment was conducted by the Master in Data and Design program in collaboration with Elisava in Spain and the Design Research Institute at Chiba University in Japan. The experiment aimed to explore the potential of generative models in cross-cultural contexts and to identify ways to mitigate their biases.
This specific experiment was done by a team of students: Thinh Truong, Bannawitit Jitchoo, and Andy Di Leo in fours days, so bear in mind that the quality of the end results is relatively low, but already shows the potential of this technique for future, more resourced experiments.
To do so, we selected an apparently innocent cultural artifact such as a toy, specifically one of the most iconically globalized toys: Barbie and Ken. Barbie dolls have sold around 1 billion since they started selling toys half a century ago, making Barbie one of the highest-selling toys ever, alongside Hot Wheels, Rubik’s Cube, and Cabbage Patch Dolls.
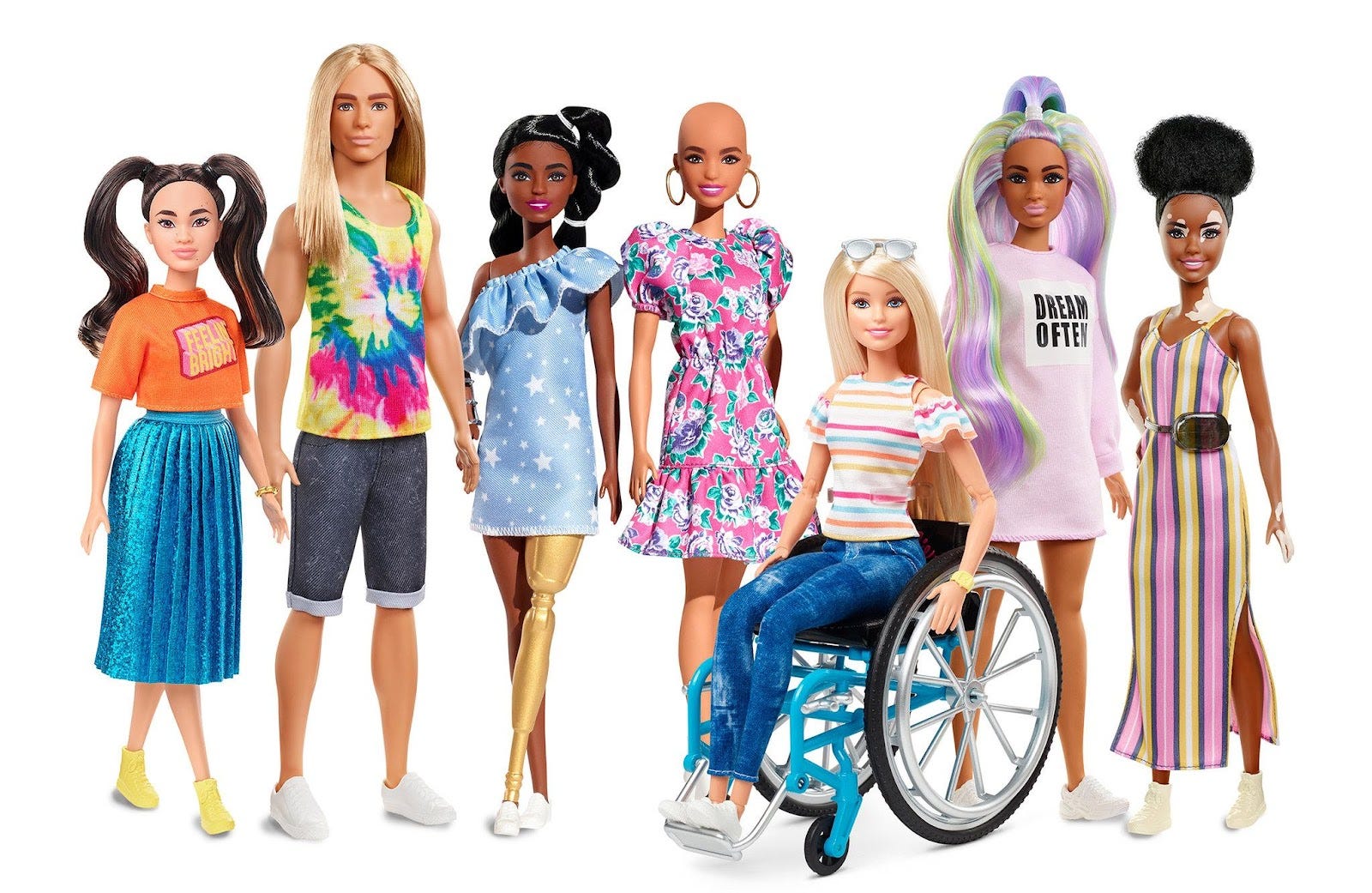
For this article, we explored how we could retrain the Stable Diffusion model (the biggest open-source generative model) with images from different ethnic groups. We selected communities that have faced historical marginalization and discrimination from dominant cultural groups or governments and have subsequently struggled for recognition, rights, and cultural preservation. The aim was to hack the algorithm to crossover concepts and representations between massive global phenomena such as Barbie Dolls with communities that have been historically underrepresented . The first selection included images from: AKHA, Tailue, Longneckkaren, Kachin, Phutai, Taiyai, Lisu, Dara Ang, Brau, Co Tu, Dao Do, H’mong, Pa Then, Xa Pho, Lo Lo, Phu La, San Diu, Ha Nhi Den, Inuit communities.
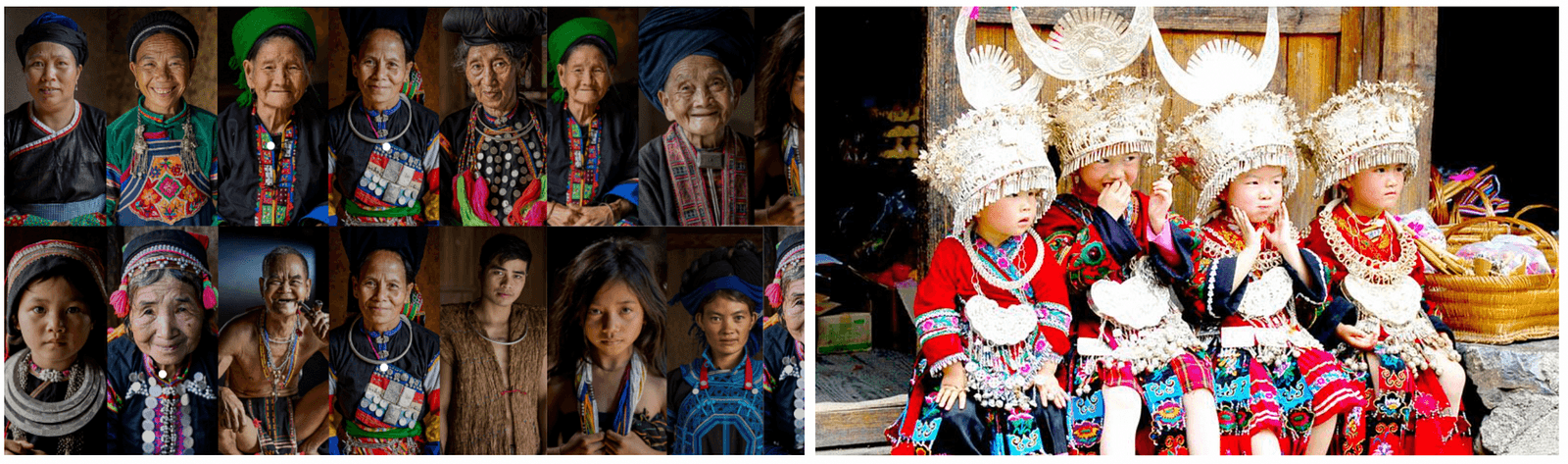
A small Database of over 100 images of dolls and ethnic community representation was fed. The selection of the pictures would determine how the generated output would look, and in this case, we aimed for a frontal product shot of the dolls. Thus, we only chose the full-body frontal pictures of Barbies and Kens on a white background. The image selection was made from creative commons and published media databases where the subjects stood out from the surroundings.
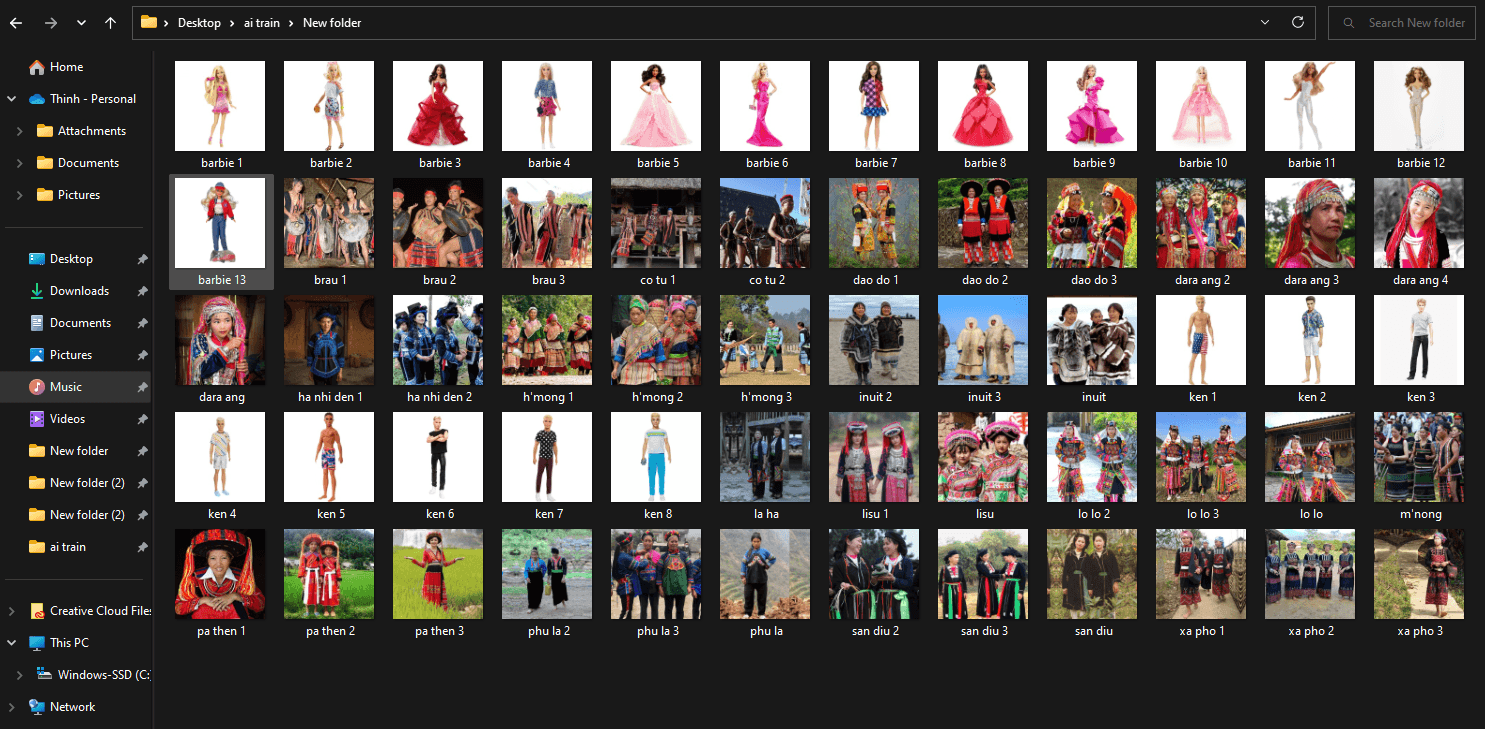
Once we had the images, we had to tag each one individually, assigning a ‘caption.’ This process would normally be carried out with a reverse prompting technique, feeding the AI a folder of images and automatically generating the corresponding captions for all the pictures. However, in this case, it wouldn’t work, as most of the images were deemed unrecognizable as they bore no resemblance to any of those featured in the original Stable Diffusion database. That meant switching to a more manual way of pairing between image and caption edits. This highlights the fact that these models lack certain representations in their datasets and demonstrated how this model was incapable of correctly identifying the selected ethnic groups.
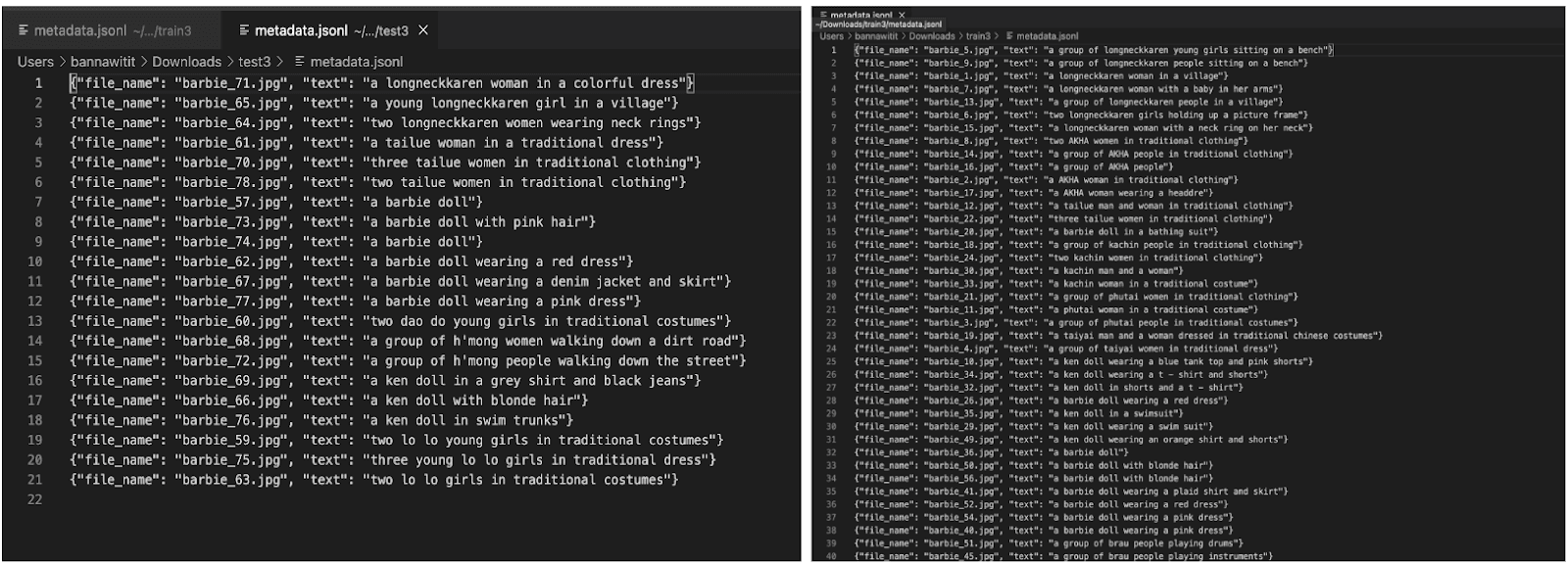
From there, we started to use tailor-made prompts to build up images; these are some of the results:
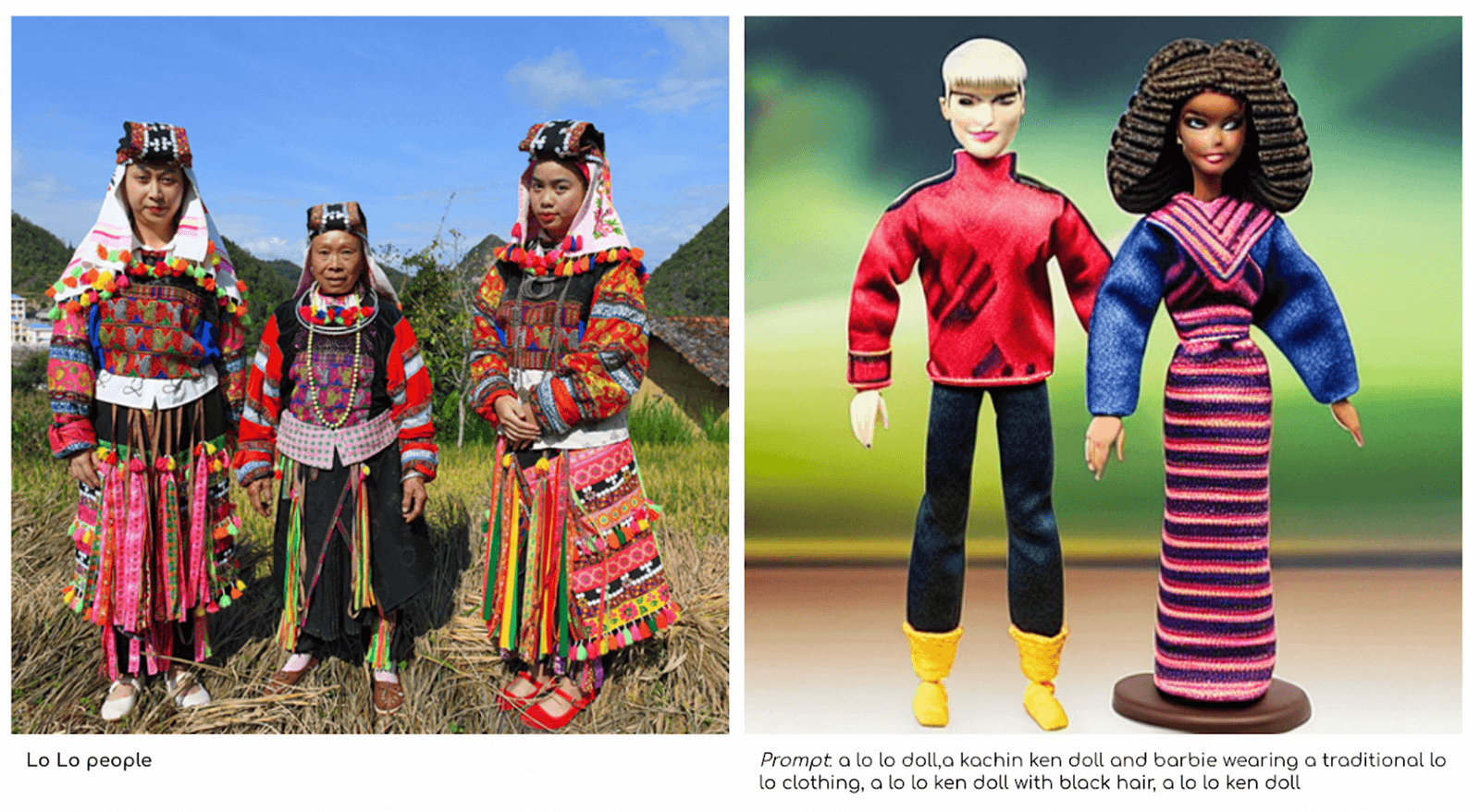
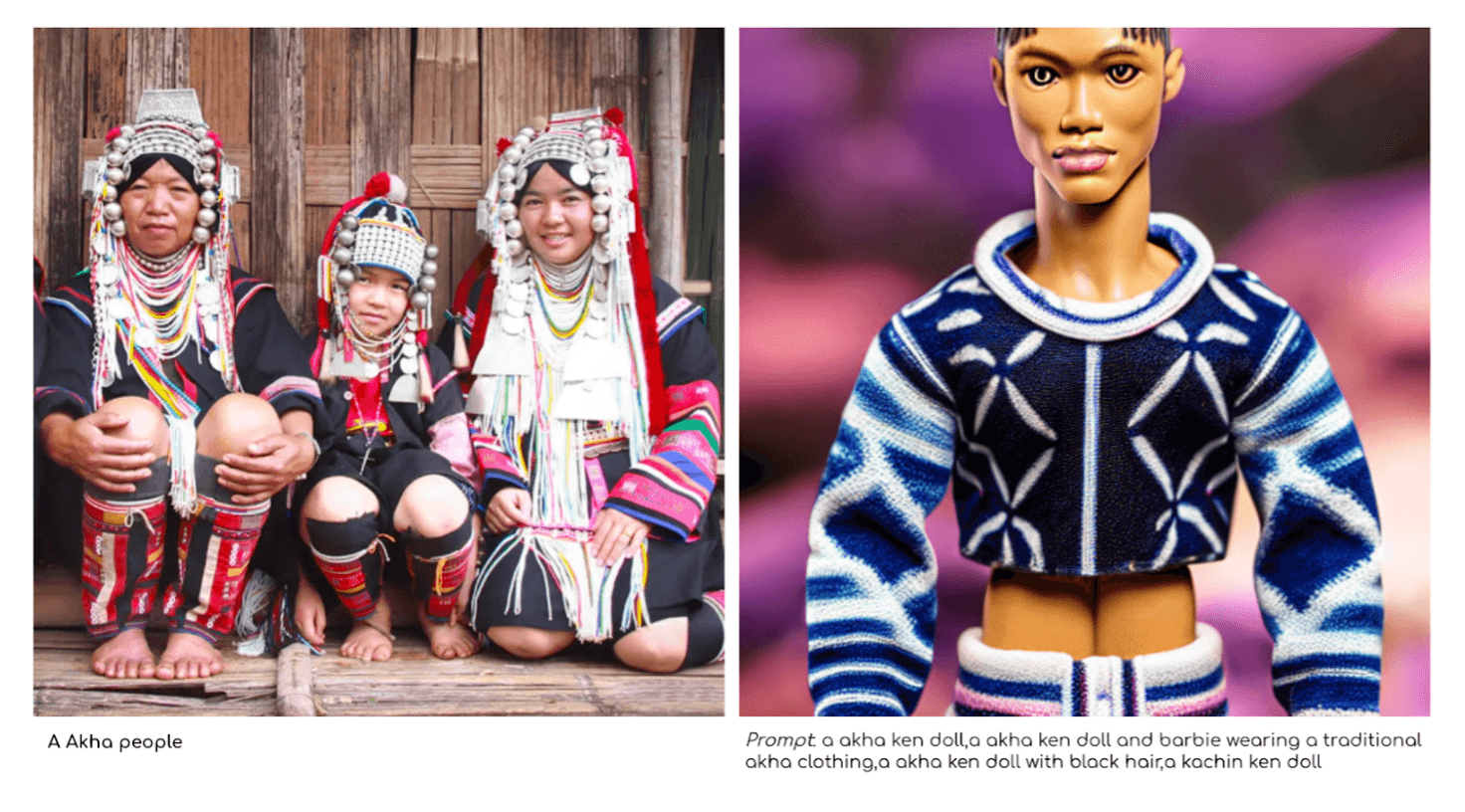
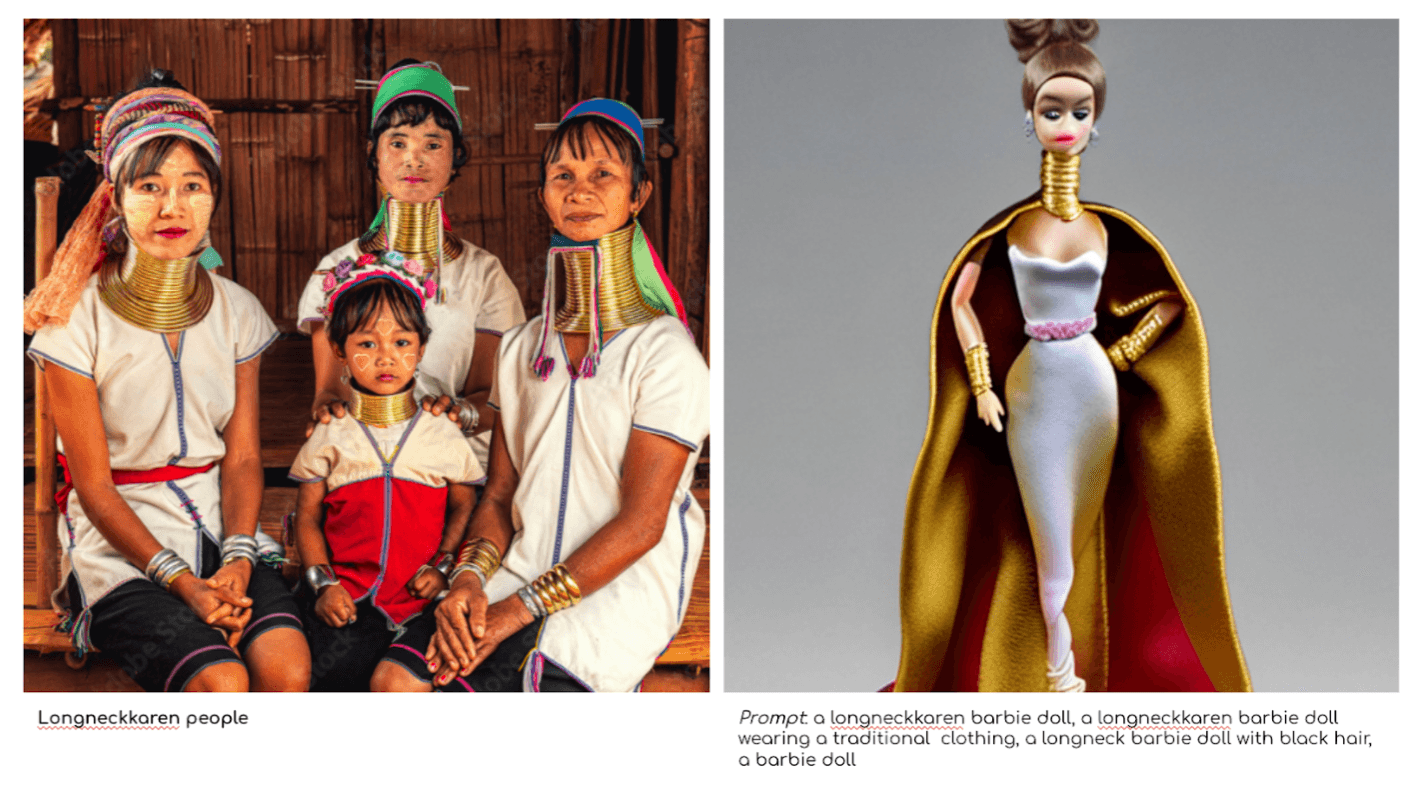
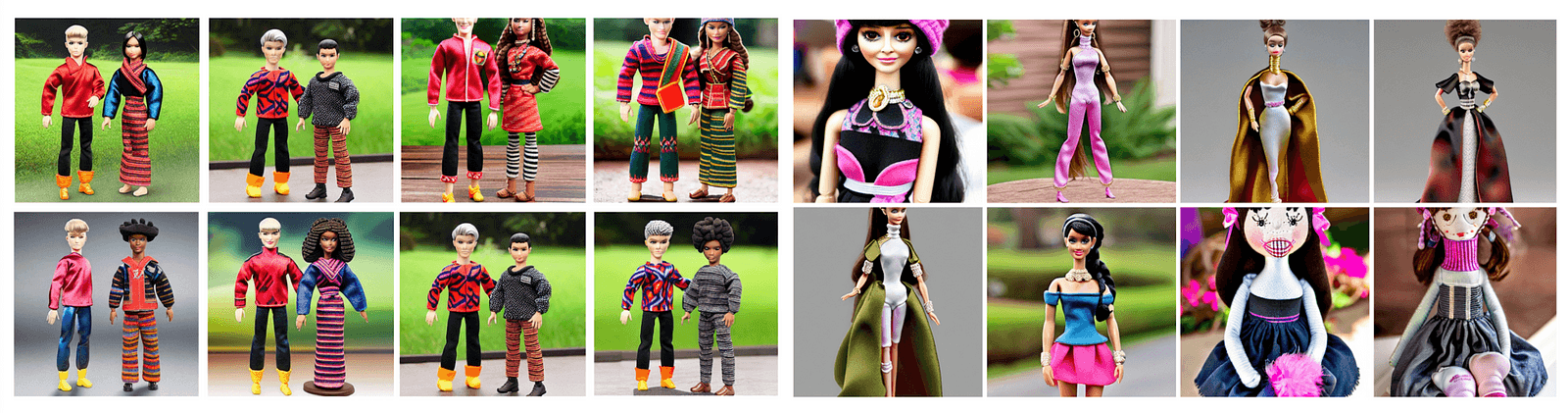
The AI race of tech companies is concerned with finding the simplest and fastest algorithms to capitalize on data. Information compression produces the maximum profit in corporate AI, but from a social perspective, it produces higher discrimination and a loss of cultural diversity.
We should encourage the creation of tailor-made DIY models, where the distinct characteristics of each community can emerge without being overshadowed by an algorithmic average controlled by very specific corporate systems.
This is an experiment but also an open path, a way to point out that whilst we become more efficient at using generative AI, we should be putting just as much time and effort into the creation of alternative models that may better represent who we are, and that can define alternative values, realities, and unique perspectives.
The final questions to raise will be: How can we facilitate the production of more diverse data sets? How can we protect the accessibility to the corporate AI database?
— — — — — — — — — — — — — — — — — — — — — — — — — — — — — — — — — — — — — —
If you are more interested in cases, lectures, and talks about AI, ping us up at hello@domesticstreamers.com
Special thanks to the amazing teachers at Chiba University, Hisa Nimi and Juan Carlos Chacon, for making this collaboration at the Master of Data & Design possible.